Introducing the Inference Economy: Spectral's Machine Intelligence Network
Bridging the gap between machine learning and web3 through verifiable, trustless inference feeds that can be streamed directly into smart contracts.
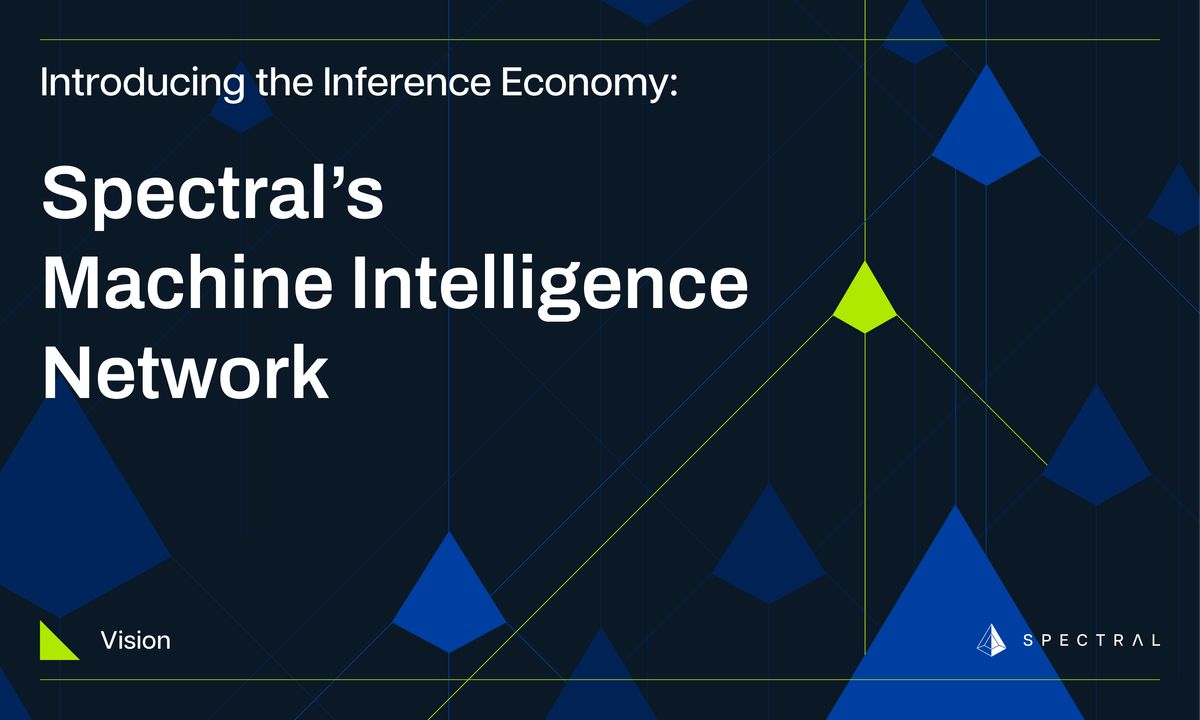
Machine Learning for web3 is broken; zkML offers a solution
Most machine learning (ML) models used in production for critical use cases are built by a handful of large, centralized players using proprietary model training techniques. They’re black boxes. Using them in a smart contract means relying on a single source of truth and creating a single point of failure.
As the demand for onchain artificial intelligence (AI) grows, so does the need for a system that can bridge ML inferences on-chain. Zero-knowledge machine learning (zkML), a recently developed suite of cryptographic technologies, can prove that an ML inference originated from a particular model.
To deliver on this demand, we’ve developed a Machine Intelligence Network, powered by Spectral’s unique validation mechanism in addition to zkML that can bridge the gap between ML and smart contracts, and usher in what we’re calling the Inference Economy: an incentivized system that rewards machine intelligence miners, protects their intellectual property (IP), and generates ML inference feeds to be consumed directly by smart contracts.
Streaming inference feeds make ML verifiable and trustless
While existing oracle networks provide raw data feeds, most notably for price data, Spectral offers feeds of high-quality ML inferences that are ready to be used onchain.
Our Machine Intelligence Network operates as a dynamic two-sided marketplace, generating high-quality inferences. On one side are miners (Modelers) who train and contribute ML models in a privacy-preserving manner. On the other, are Consumers who demand custom, high-quality inferences.
Our core product is inference feeds, streamed directly into smart contracts, which offer Consumers a composable, decentralized, and high-performance way of consuming ML.
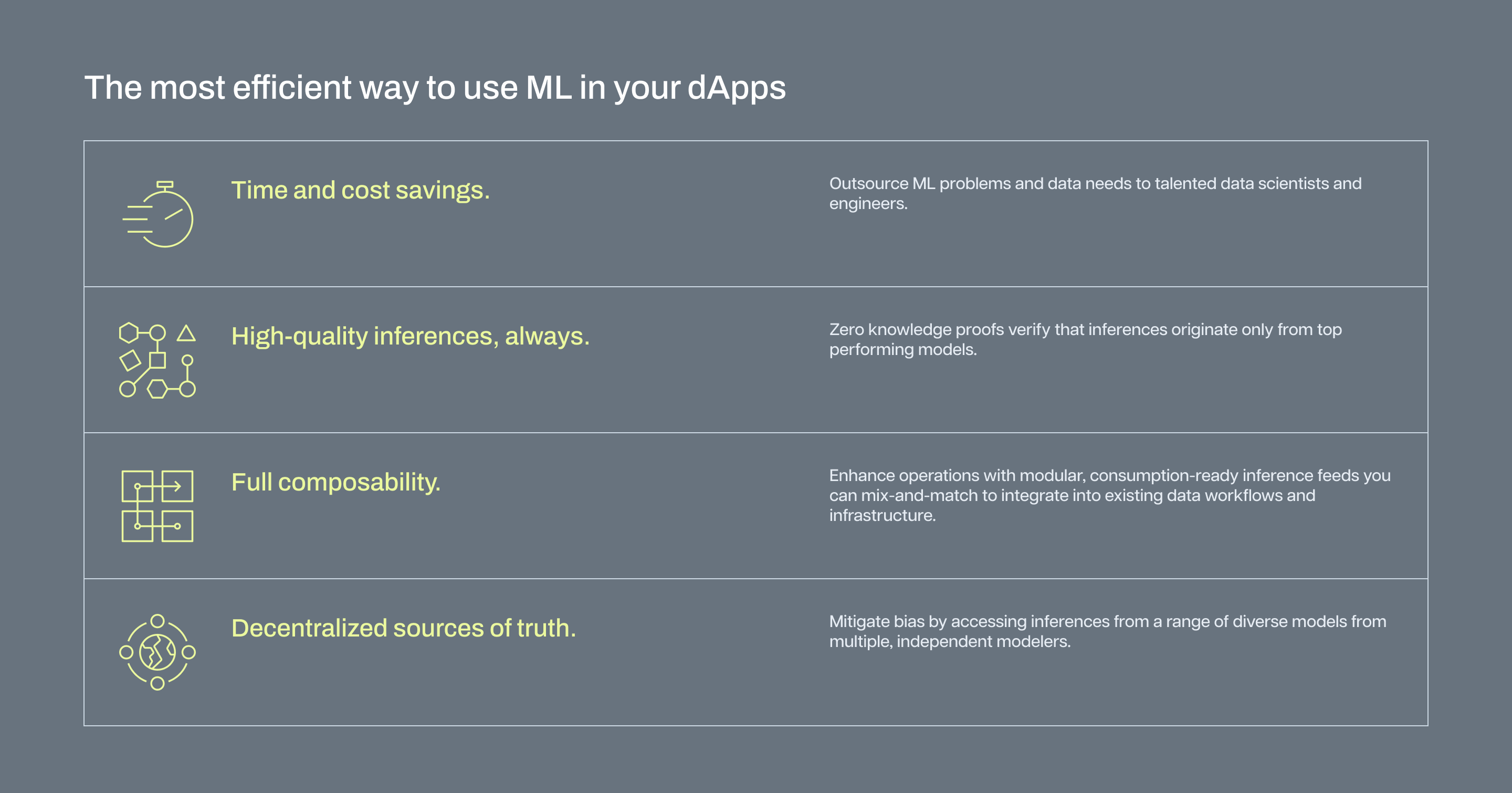
There are four key benefits to using this system: it’s faster to outsource ML problems than it is to recruit and train a team of in-house experts. Inferences are constantly verified by zkML, ensuring that they’re not faulty. We also ensure they meet performance benchmarks through Spectral’s unique validation process. They are also fully composable, allowing them to be used in smart contracts or a myriad of other applications, and finally, by drawing expertise from a diverse and dynamic group of machine learning experts, they help avoid common pitfalls such as bias.
We’re changing how web3 perceives and interacts with machine intelligence
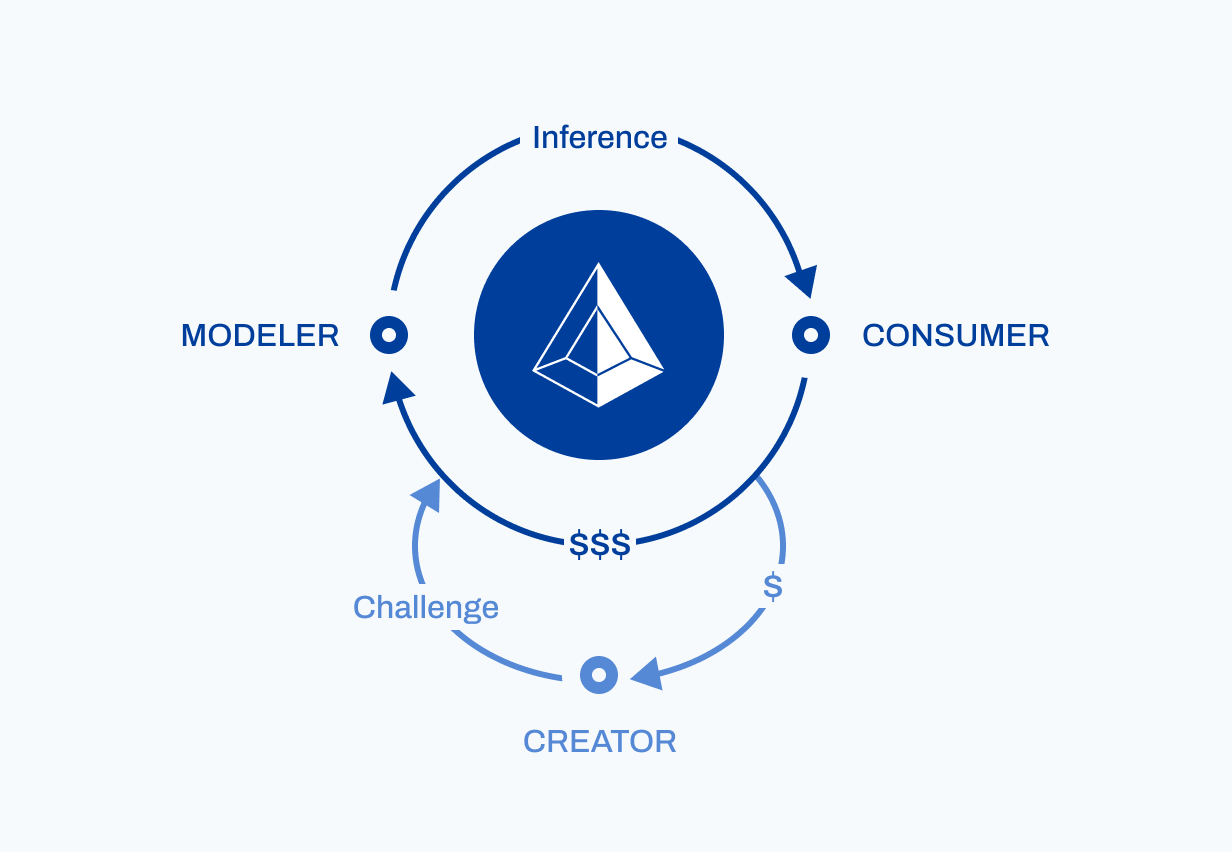
There are four players in Spectral’s network:
Creators are web3 companies that post data science/ML challenges, set performance benchmarks, and establish rewards for winning Modelers. They earn a share of the revenue generated by the consumption of inferences from their challenges. As Creators continue to post challenges relevant to other web3 companies, they receive an increasing share of ongoing revenue.
Modelers solve data science/ML challenges posted by Creators, with the opportunity to win a bounty and receive the majority of revenues from ongoing use of their model inferences by Consumers. As Modelers accumulate more rewards from high-performing models, they are incentivized to continue building better models across various challenges. Throughout the process, their intellectual property is preserved which makes perpetual incentivization possible.
Consumers discover inference feeds that align with their data science/ML needs and pay fees to access them. They can harness the power of Spectral's decentralized community to obtain permissionlessly built models that produce readily ingestible high-quality inferences, all at a lower cost than developing models in-house.
Validators ensure models do what they claim to be doing, in terms of both integrity and quality. During the competition, they use a randomness beacon to create a unique random test set for each Modeler that they upload to IPFS. After the competition, Validators check Modeler responses against ground truth, verify zkML proofs, and ensure performance benchmarks set by the Creator are hit.
This enables a flywheel, we depart from the status quo of ML (where a handful of large, centralized players produce important inferences). Instead, we usher in a new era: one where any agent (smart contracts, LLM, etc.) on the Spectral network can automatically verify, rather than merely trust, inferences and outputs from other ML models and use them readily in their applications.
Spectral unlocks the potential of web3 and AI
Combining web3 technologies with AI and ML can unlock enormous potential, but the connection between the two has to be made.
As web3 increasingly intersects with traditional institutions, the need for transparent and reliable AI will rise. That demand is further amplified by recent events that highlight the importance of accurate and verifiable inferences, such as a major credit bureau's mishandling of millions of credit scores last year. Web3's core principles of transparency and decentralization offer a promising solution to ensure safety and reliability in AI applications. Bridging the gap between these principles and AI usage is essential.
At Spectral, we're addressing this challenge by integrating advanced concepts like zkML, enabling the creation of an Inference Economy that will bring AI and machine learning inferences onto the blockchain, helping organizations broaden the scope and capabilities of their web3 applications.