The Future of Credit Risk in Web3 — It’s Not Just a Credit Score

Written in collaboration with Raymond Anderson
Before 2010, credit risk assessment methodologies had been relatively stable for many years. Of late, however, gains in computing and data storage and processing efficiencies have accelerated and changed the landscape on two fronts. First, the depth and breadth of available data (“big data”). Second, the techniques used to convert it into usable information. For the latter, the advancements have predominantly been led by neo and challenger credit institutions. The big players, i.e. credit bureaus and lending institutions, were mostly resistant to this change — at least at a sufficiently large scale. Their experimentation and use of alternative methodologies are much more recent.
Even more recently, Web 3.0, the Ethereum blockchain, and its smart contracts have been redefining the financial landscape. They have enabled decentralized finance (DeFi), which has near-instantaneous loan processing times, round-the-clock availability, and the absence of a central regulatory body or intermediary. Despite the rapid advancements, it still lacks a robust credit risk assessment mechanism, largely because it is not possible to identify human or corporate counterparties. As a result, any borrowing is currently heavily over-collateralized.
Drawbacks of Current Credit Risk Methodologies
But why should we even support this transition? The inefficiencies of existing credit risk mechanisms were made apparent by shocks like the Global Financial Crisis and the Covid-19 pandemic — latter, albeit not at the same scale. Despite having access to humongous amounts of new data, the credit infrastructure at many institutions remains rigid. Links to source systems are complex and data quality is problematic (inaccurate, incomplete, dated, etc.). This inflexibility is usually caused by a multitude of data integration/ingestion pipelines, various processing environments, and several data sources — all potentially competing with each other together with an inefficient evolution cycle over decades.
Another inhibiting factor is changed requirements set by central banks and other regulatory authorities. These requirements are often very stringent and inflexible, leaving minimal room for innovation or transformation. Regulators often have a poor understanding of emerging technologies; and, are generally resistant to significant and rapid overhauls of credit risk systems to avoid substantial shifts in credit risk distributions. Points-based models developed using traditional techniques have been favored over more modern techniques that can provide better results but are less transparent.
Credit scoring, as is prevalent now, remains siloed and deeply centralized within a core set of players, e.g., FICO, Equifax, Experian, TransUnion, etc. Credit and lending institutions usually add their own layer of policy rules on top of the data received from these independent providers. Known as the banks’ Internal Risk or Rating Systems (IRS), Jacobson et al. 2007 noted that each bank may have its own different lending or risk management styles with each one of them striking their own balance between risk-taking and (the cost of) monitoring (that risk). However, the centralized nature of credit risk assessments still holds — it involves trusting centralized credit bureaus with personal and confidential information. Concerns around data privacy and security have been extensively researched, reported, and commented upon, especially since the 2017 Equifax data breach.
Related to the issue of centralization is the fact that the majority of credit scoring methodologies have never been made fully public — and remain more or less opaque. Although all credit rating agencies are mandatorily required to fully disclose their credit rating methodologies to the public, the same does not hold for credit bureaus. They instead disclose the high-level factors that are likely to impact or support an individual’s credit score, e.g.:
- Loan repayment history — has a borrower ever missed a payment or defaulted on a loan?
- Value of outstanding loans (usually considered relative to one’s income) — can a borrower service an additional loan?
- Credit utilization — the proportion of credit lines utilized by a borrower and its historical trend
- The number of open and closed accounts or credit lines
- The number of credit inquiries — is the potential borrower shopping for credit, which can indicate credit distress?
- Length of credit history — longer credit histories generally reflect positively on credit scores
Alternative Credit Data
Of course, most of the above factors apply only to current and historical borrowers — the “financially-included”. No due consideration is given to non-borrowers, except to the extent that rental and other contractual payments may be included by some (where available). As a result, credit participation for un- and under-banked population is limited, at least for those who would otherwise be reasonably creditworthy. The Global Findex database, produced by the World Bank, reported in its 2017 edition that 69% of the global adult population — 3.8 billion people — had a bank account, with 1.7 billion remaining unbanked. This represents a substantial chunk of the market that remains untapped. Several neo and challenger credit institutions, e.g., CredoLab, Kabbage, LenddoEFL, Credit Kudos, etc., have started incorporating alternative data as part of their credit assessments to counter this financial exclusion.
The rise of alternative credit providers has somewhat supported the inclusion of alternative data in credit risk assessments. A working paper published by the Bank For International Settlements (BIS) in September 2020 (Cornelli et al., 2020) estimated that fintech (online market places bringing together borrowers and lenders) credit volumes reached USD 297 billion in 2018, while big tech credit (large firms with multiple business lines, of which lending represents only one part) volumes surged to USD 397 billion. This represents a dramatic increase since 2013, when volumes were only USD 9.9 billion and 10.6 billion, respectively. While still small overall, fintech and big tech lending flows are much larger relative to the overall financial lending volumes in certain economies, notably China and Kenya.

A recent study by the Federal Reserve Bank of Philadelphia’s Consumer Finance Institute and authored by Jagtiani et al., 2019 into the loans originated by LendingClub, a large fintech lender, found “a high correlation with interest rate spreads, LendingClub rating grades, and loan performance”. The study further indicated that “nontraditional alternative data have been increasingly used by fintech lenders” and that “the use of alternative data has allowed some borrowers who would have been classified as subprime by traditional criteria to be slotted into “better” loan grades, which allowed them to get lower-priced credit. In addition, for the same risk of default, consumers pay smaller spreads on loans from LendingClub than from credit card borrowing”.
J. Jiang et al, 2021 noted that utilizing alternative data as part of credit scoring predicts an individual’s likelihood of defaulting on a loan with 18.4% greater accuracy than the lender’s internal score. Moreover, the impact of the big data credit score is more significant when evaluating borrowers without public credit records.
Evidence from a 2017 regulatory shock in China (Gambacorta et al., 2019) suggests that models with alternative data and machine learning may outperform traditional data in predicting distress after a shock.
Examples of such alternative data include:
- Income and employment information
- Rent, utilities, cable, mobile, and other regular bill payments — does an individual pay them on time and in full?
- Savings/Checking account balance and trend thereof, often referred to as positive credit data
- Purchasing pattern/behavior — does an individual indulge in discretionary expenditures?
- Asset ownership details
- Social media
It is worth noting that some of the traditional credit bureaus have also started offering credit scores based on alternative data, e.g., FICO Score XD, UltraFICO, and Experian Boost, predominantly through acquisitions and/or partnerships; however, they have not been widely adopted yet by the large financial institutions.
Credit Risk in Web 3.0
Web 3.0 has been envisioned as the decentralized internet for the future: backed by open source applications, absence of a central body to regulate online activity, and being inherently trustless, i.e., all participants make financial transactions without requiring a central trustworthy third party.
DeFi borrowings are inherently a lot more fluid than the traditional finance borrowings — multiple loans may be made with no fixed repayment schedule, all of which can then be repaid at once or through multiple repayments of varying amounts over an extended period. To compensate for the lack of KYC information and effective credit risk mechanisms, DeFi borrowings currently are heavily collateralized (up to 150 percent in some instances), a situation that is arguably highly capital-inefficient, favoring the affluent over the marginalized population. Further, the collateral can be immediately liquidated should the borrowing increase or collateral values fall such that the collateral ratio dips below the prespecified threshold. As a result, borrowers are highly conservative with their borrowings.
The Total Value Locked (TVL), which represents the total amount staked with DeFi protocols, has increased by over 5 times in just the last one year and currently stands at USD 92 billion. The top DeFi protocols include Aave, Maker, and Compound (excluding Instadapp which acts as a bridge between various DeFi protocols). Total debt outstanding across all DeFi protocols witnessed an even sharper growth of over 13 times, currently at USD 22 billion.
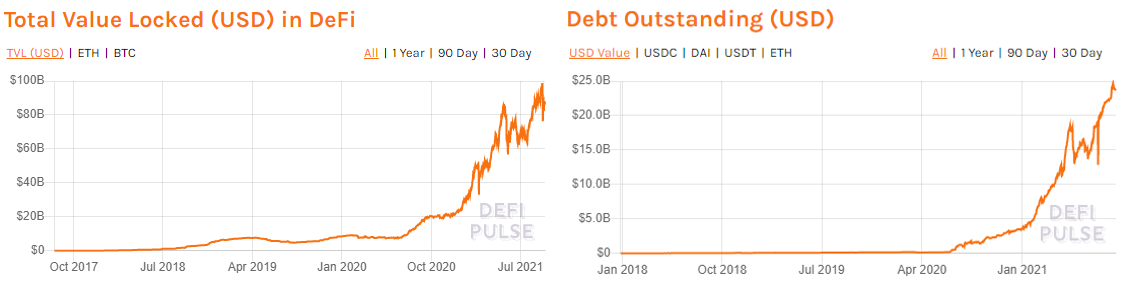
Based on the data compiled by Richard Chen using Dune Analytics, which tracks unique addresses interacting with major DeFi protocols, there were 3.3 million DeFi users at the end of August 2021 — up from 425k a year ago. Note that these are counts of unique addresses and can have some over-representation since a single person can own multiple addresses at the same time.

Spectral & Programmable Creditworthiness
We at Spectral Finance propose a paradigm shift to the DeFi lending ecosystem by introducing credit risk infrastructure based on a user’s on-chain activity and behavior. The inarguably natural evolution towards credit scoring aligns with the premise of Web 3.0: the absence of a central scoring body and ease of access to anonymous data that can be tied to an individual’s credit risk. There have, however, been minimal efforts to date to assess an entity’s credit risk using data that is both decentralized and anonymous.
There is a wealth of information relating to a user’s on-chain activity that can only grow over time, to reflect an aspirant borrower’s risk appetite and the inherent credit risk. One must note, however, that for DeFi the concept of “default” is replaced by “liquidation”. In traditional credit, basic credit risk models provide a ‘probability of default’, which is an event indicating an elevated probability of non-payment, potential bankruptcy, and liquidation. We lack that marker, so instead provide a probability of liquidation directly using on-chain behavioral data. Considering a distress event higher up in the severity chain mitigates the perceived higher risk of interacting with anonymous addresses on DeFi and Web 3.0.
Some of the on-chain data points that can be used for credit risk assessment include:
- Number and volume of the following transaction types, including various time-based related metrics:
- Borrowing
- Repayment
- Deposit/Lending
- Redemptions
- Liquidations
- Credit mix — diversity of interactions with various DeFi protocols
- Wallet transactions, including wallet balance
- Account health-related indicators, e.g., the amount borrowed as a proportion of the available collateral
Most of the above, highly critical and informative yet unprecedented data points, are closely related to the traditional credit risk factors, allowing a level of comfort on DeFi credit scoring. It is our aim to employ Machine Learning techniques to steer towards automated credit risk modeling, which lends itself to DeFi and Web 3.0 and is a far cry from traditional methods that combine scores with policies and manual overrides and are potentially more error-prone.
We are well aware of particular challenges to DeFi credit scoring (potentially incomplete on-chain activity pattern of a natural person, bias risk, etc.). Irrespective, we truly believe in its full potential once it is properly harnessed through disruption at the base layer in how credit risk is formulated, implemented, and makes way for programmable creditworthiness. Our plan is to gradually and iteratively transition towards a more efficient DeFi lending ecosystem while effectively managing and mitigating such challenges.
Our roadmap includes the following to engender trust and reliance on credit scoring:
- Programmable creditworthiness — Spectral lays the framework for a new primitive: on-chain transactional history as a composable asset.
- Verifiable Computation & Zero-Knowledge proofs, where proofs of a (machine learning) model’s claims will be published alongside credit scores so that users don’t need to trust a single specific entity fully
- Distributed credit risk modeling, where credit scores developed by different entities through alternative models can be aggregated to provide a more robust and holistic credit score
- Reward and incentive mechanisms to encourage users to bundle together their Ethereum wallet addresses to allow a holistic credit risk assessment
Spectral’s vision of DeFi lending is to engender trust among DeFi participants through democratized and decentralized credit risk assessments leading to a more capital-efficient credit landscape where credit risk assessment is composable across the entire Ethereum ecosystem.
References
Cornelli, G., Frost, J., Gambacorta, L., Rau, R., Wardrop, R. & Ziegler, T. (2020). FinTech and big tech credit: a new database. BIS Working Papers No 887.
Gambacorta, L., Y. Huang, H. Qiu and J. Wang (2019): “How do machine learning and nontraditional data affect credit scoring? New evidence from a Chinese fintech firm”. BIS Working Papers, no 834
J. Jiang, L. Liao, X. Lu, Z. Wang & H. Xiang (2021). Deciphering big data in consumer credit evaluation. Journal of Empirical Finance, Vol 62.
Jacobson, T., Lindé, J. & Roszbach, K. (2006). Internal ratings systems, implied credit risk and the consistency of banks’ risk classification policies. Journal of Banking & Finance, Volume 30, Issue 7.
Jagtiani, J.A., & Lemieux, C. (2019). The Roles of Alternative Data and Machine Learning in Fintech Lending: Evidence from the Lendingclub Consumer Platform. FRB-Philadelphia: Working Papers (Topic).