The Art and Science of Credit Scoring
Part three of the History of Credit, Credit Intelligence, and Credit Scoring which charts the gradual development of scoring and how it spread, eventually contributing to the credit crisis of 2009. Decentralized scoring is proposed as a potential solution.
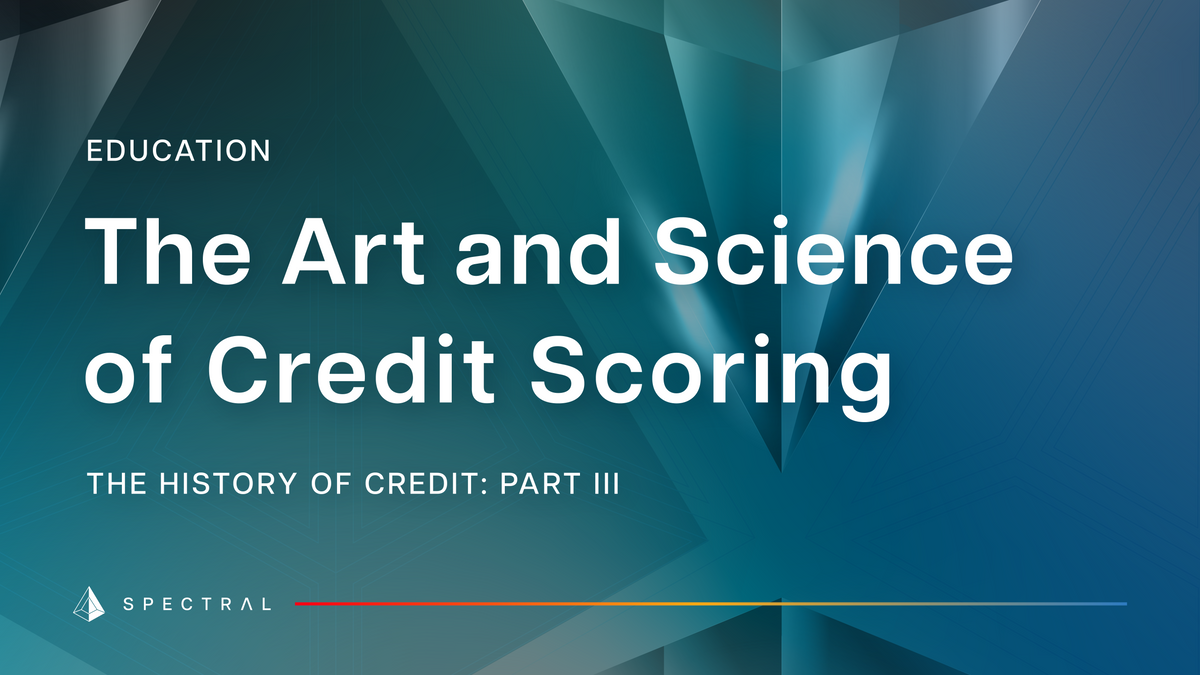
The conclusion of our series on the History of Credit, Credit Intelligence, and Credit Scoring. This essay traces the development and application of mathematical methods like linear programming and discriminant analysis and the technological and regulatory forces that led to mathematical credit scoring. We conclude with a vision of the future and discuss how decentralized identities and on-chain credit scoring could solve some of the privacy issues created by centralized credit intelligence while paving the way for new products and marketplaces. We recommend reading Part 1 and Part 2 first.
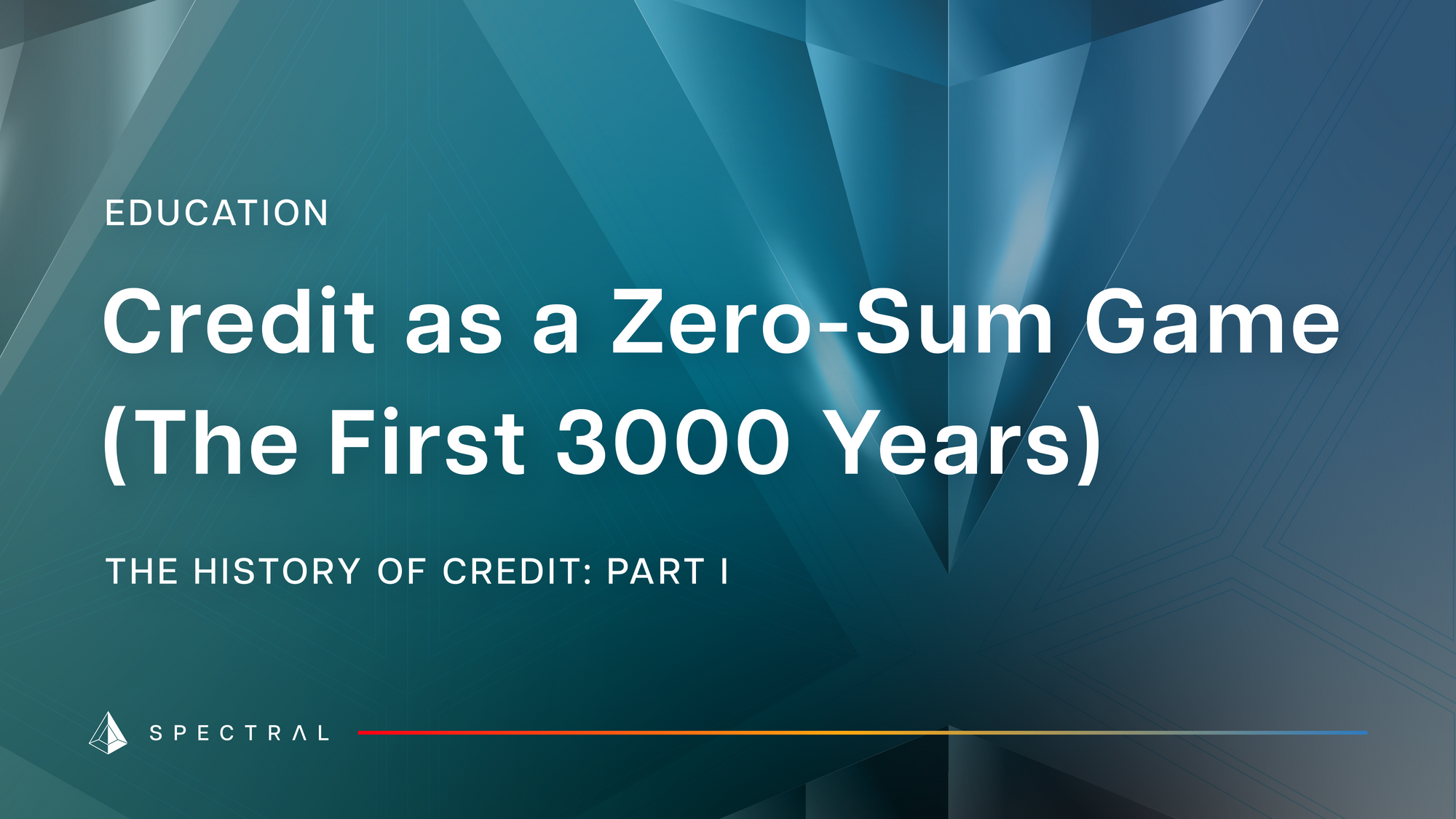
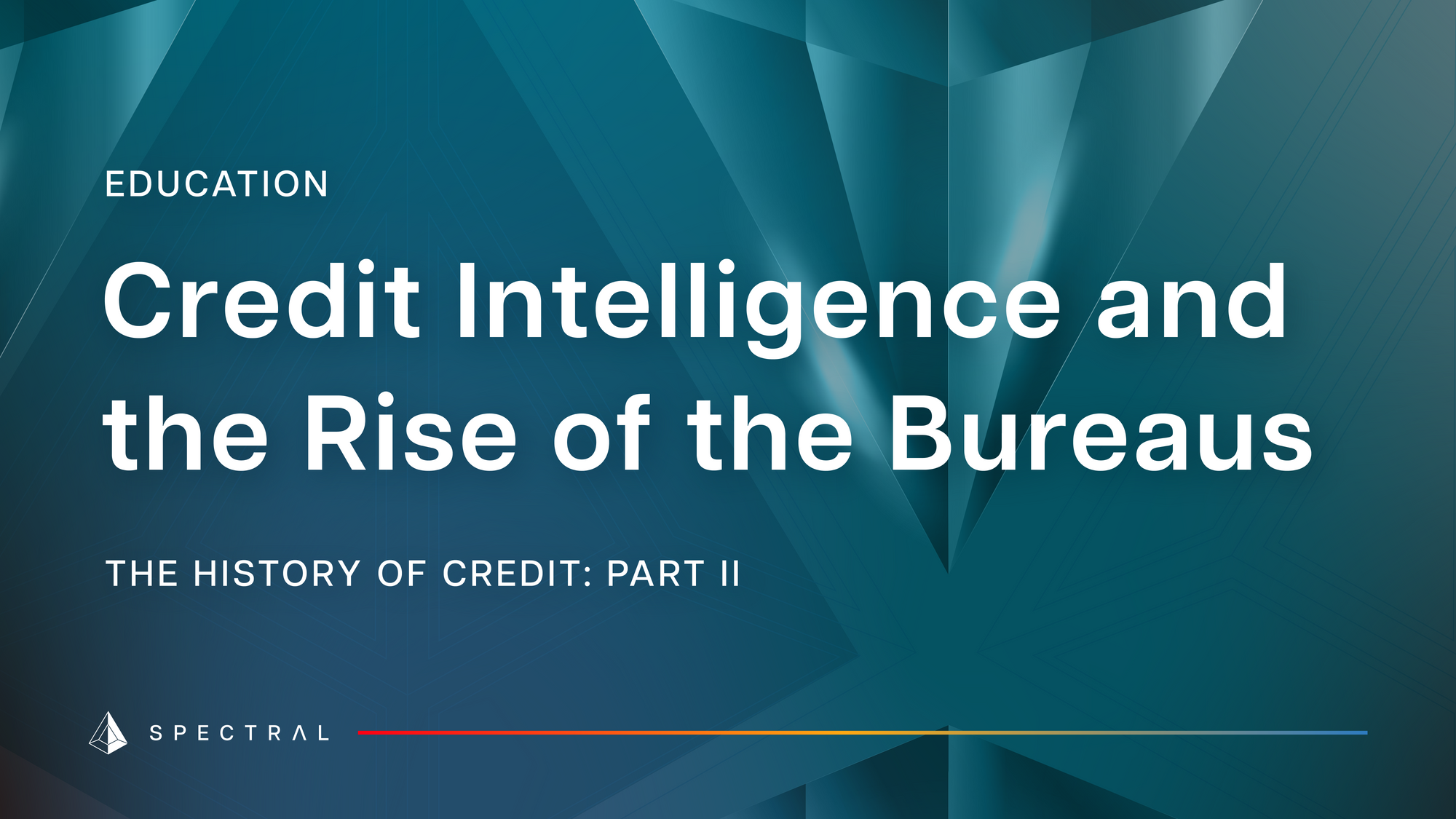
For millennia creditors were forced to rely on the three Cs of credit—character, capacity, and capital–to make their lending decisions. There were a few attempts at codification and clarification: in the 19th century, Dun & Bradstreet began assigning grades, but there was little science behind those grades. As consumer credit expanded, credit men and women remained the lynchpin of the credit system. If a customer didn’t pass muster with them, then all the documentation in the world couldn’t help.
Empiricism was beginning to take hold. In the 1930s, credit men began looking at actuarial science, thinking about how insurance providers used demographic data to price life insurance. “The first known use of scoring was for mail order,” writes Raymond Anderson in Credit Intelligence and Modelling (282) “Spiegel implemented a ‘pointing system’ using five demographic variables—including occupation, marital status, location, and race.” Sampling pioneer David Durand also identified a Phoenix bank that used a score based on customers' financial records, income statements, financial statements, the type of security being borrowed, and past payment performance to assess applicants. World War II increased the pressure as credit men were called to serve, and “before they left, they codified their rules of thumb for use by housewives in employed in their stead for the war’s duration.”
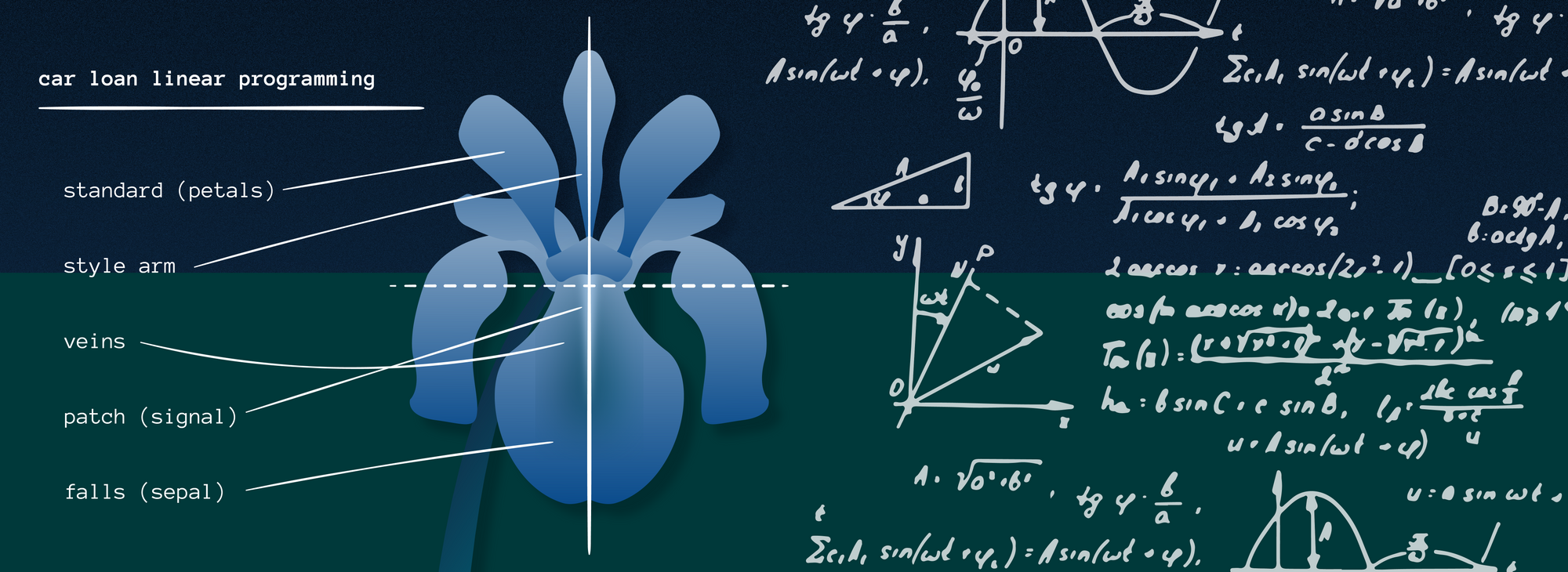
The 1930s also saw the development of linear discriminant analysis (LDA) by Sir Roland Aylmer Fisher. Fisher needed a method of classifying three iris species based on their sepal length and width and the length of their petals. David Durand took LDA and, in “Risk Elements of Consumer Instalment Financing” (1941) applied it to a set of 7,000 successful and unsuccessful car loans obtained from banks and finance houses (284) to see whether actuarial practices could be adapted to credit decisions. Durand, working for the National Bureau of Economic Research, polled credit executives to determine which factors they considered most essential and used LDA to determine how much of a correlation each factor had on the outcome of the loan. “The result is almost always the same,” writes Durand, “the good loans contain a large percentage of borrowers with long periods of employment than do the bad loans, and the average tenure of occupation is higher and longer among good loans than among the bad.” (4) He also found several other pertinent relationships such as stability of residence and ownership of a bank account and gender: “women appear better risks than men” not to mention the size of a downpayment. Type of occupation did not prove as helpful a metric and no consideration in the data was given to character whatsoever, “because of the difficulty of acquiring significant statistics.” It was an entirely empirical system on its face.
During the 1940s and 1950s, a variety of new tools emerged, including testing methodologies such as Eldon Wonderlic’s intelligence score (still used somewhat controversially by the National Football League) and, developed during a stint as director of personnel at the Household Finance Corporation, a Credit Guide Score “which instructed analysts on score calculation.” But, according to Anderson, Wonderlic later bemoaned that his analysts rarely trusted it. Operations and logistics challenges during the war led to other developments such as linear programming, which would “become the ur-methodology of today’s best-known scorecard vendor, FICO.” (285)
The era of FICO brought easy credit and helped fuel an economic revolution in the 1980s, 1990s, and 2000s. FICO Scores and their ilk are the apotheoses of the current system, the result of millennia of lending and more than a hundred years of credit intelligence, gathering evermore information on their consumers and rolling credit out to every possible niche. Eventually, something had to break.
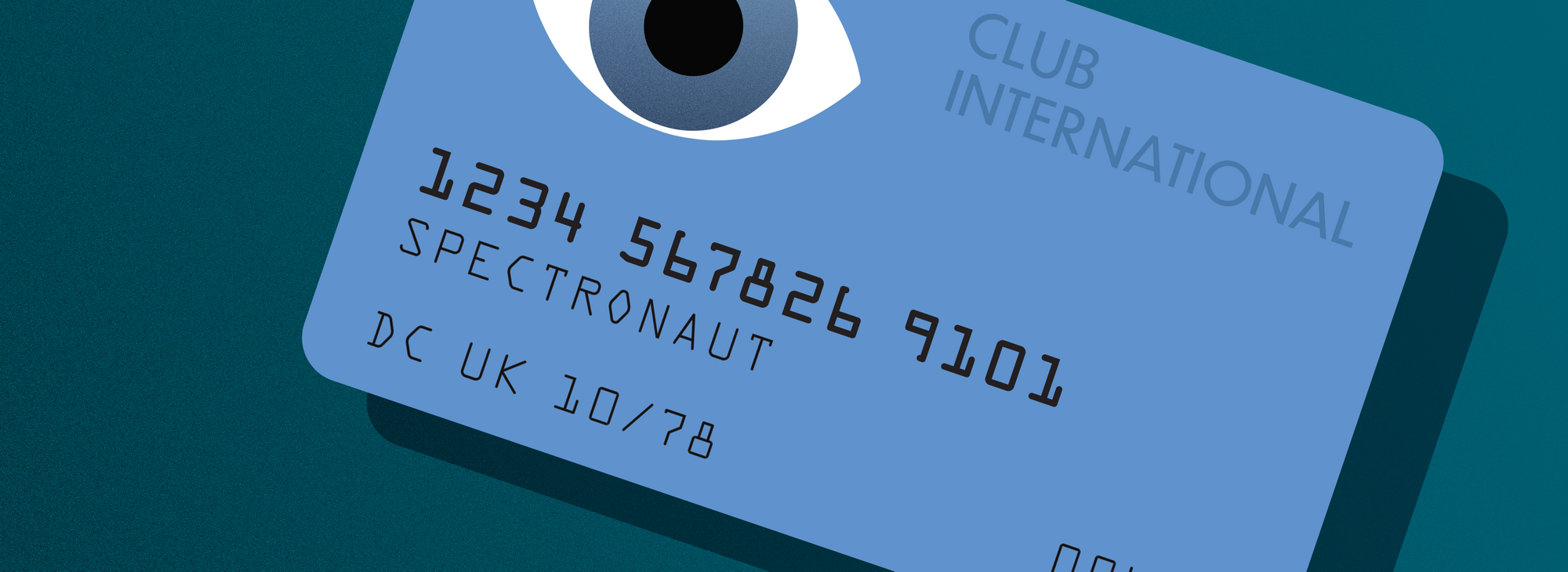
Can a deadbeat be recognized before he is granted a loan?
Or, so asked Albert Kraus of the New York Times when confronted with the FICO credit score in 1961. Four years earlier, engineer William Fair and mathematician Earl Isaac, two employees of the Stanford Research Institute (better known as SRI), were contracted by Hilton Hotels to develop a billing system for Carte Blanche, an early credit card. “They realized a predictive model could be developed using linear programming and proposed “credit scoring” to 50 prospective clients via a 1958 mailshot.” (Anderson: 286). A single company took the bait, and the following year they developed a “scorecard” for the Public Finance Company of Missouri (part of the American Investment Company). At first, the primary appeal of the score wasn’t so much that fewer customers were likely to default than ones scored by credit men and women, instead, it was processing speed. Soon the differences between AIC’s traditional installment loans and their scored ones became impossible to ignore. More companies signed up for credit scoring by Fair Isaac, including the General Electric Credit Company, which saw default rates for credit and charge cards fall by up to 50 percent. (286)
Anderson writes that uptake was slow despite the apparent advantages. Credit executives didn’t believe the algorithms worked or, given the rosy economics of the time, didn’t think loss reductions were worth the investment. Personnel shortages and the economies of scale offered by computers eventually forced the credit industry’s collective hand. Department stores were the first to sign up. Oil companies began using them for gas cards, as did charge and credit card providers in the 1960s. Early on, scorecards were tabulated by hand. Computers offered much faster decisions.
There were other forces afoot. An exploratory government committee realized the extent of the credit intelligence gathering system in the United States. By 1970, with the Fair Credit Reporting Act, American consumers were pushed back against invasive financial intelligence gathering. “A further push came in 1974 when the Equal Credit Opportunity Act was enacted against discrimination but made allowances for empirical models,” writes Anderson, referring to Fair Isaac’s scores. As the score became more broadly accepted, lenders began using it for larger and larger purchases, including car loans in South Africa in 1978 by Stannic and in 1979 by the General Motors Acceptance Corp (GM’s credit wing). Fair Isaac became a monopoly, offering scores directly to lenders. Only after a competitor, Management Decision Services (MDS), began using data from the three major credit bureaus (Equifax, Transunion, and Experian), Fair Isaac began working directly with bureaus. In 1989 FICO became the default risk assessment score for all three bureaus. In 1995, Fannie Mae and Freddy Mac, the two federal mortgage lenders (Government Sponsored Enterprises), began using FICO Scores for home loans.
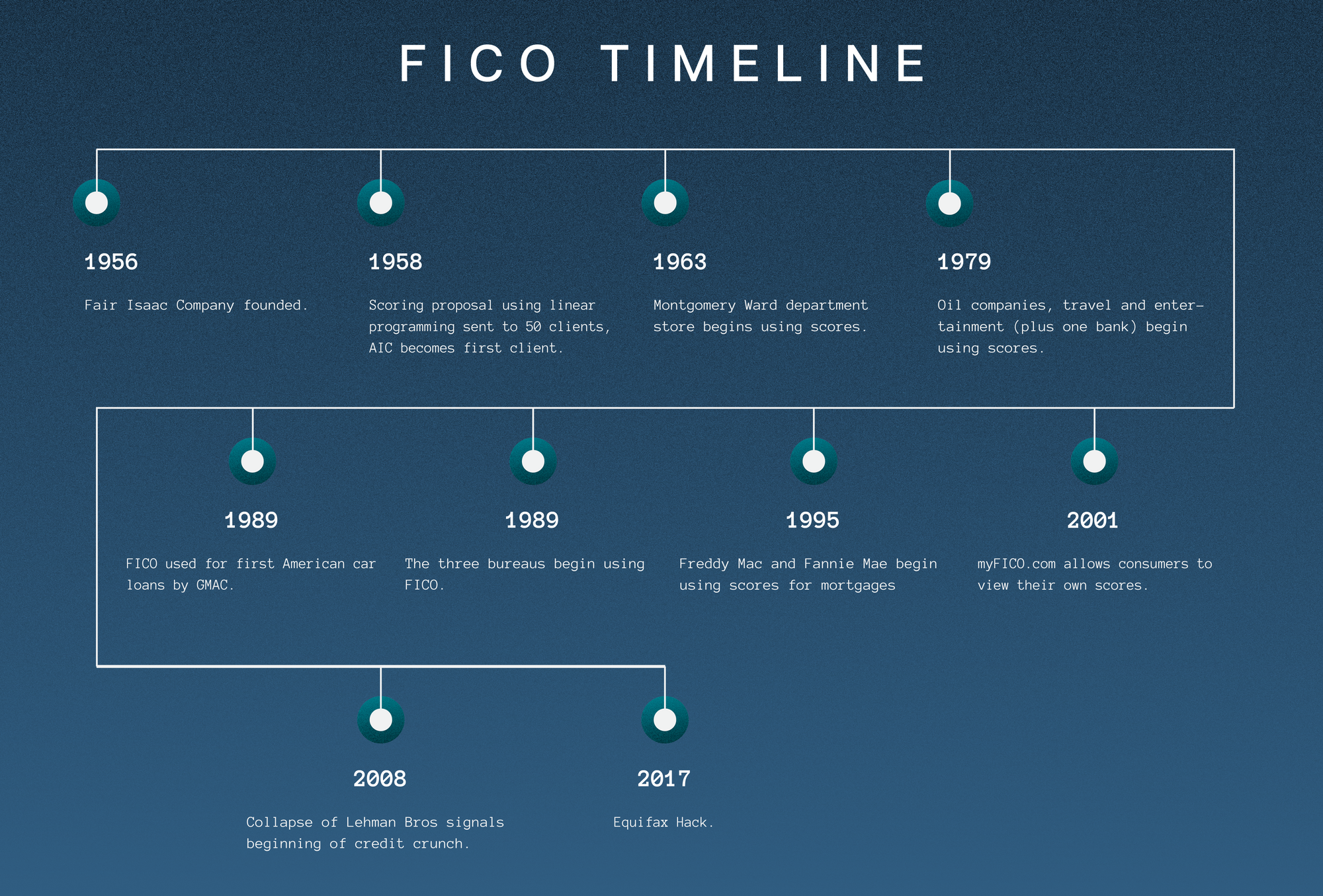
FICO is not the only score available, although it remains the largest by market share. In 2006, the “American Big Three [bureaus]” (288) created the VantageScore, which differs in that they offer bespoke models like car loan scores or mortgage scores instead of offering a single source of truth like the FICO Score. There are models that can factor alternative payment histories like medical and utility payments into their scores. Two other scores, primarily used in Europe, are Scorex and Scorelink and are similar to FICO. Various credit models have also been created to allow banks to incorporate elements of credit scoring into their derivatives and volatility models, such as RiskMetrics and CreditCalc.
The processing power and portability of FICO and its kin helped credit opportunities blossom. Household debt expanded rapidly after the development of credit cards and household mortgages. Unfortunately, there is such a thing as too much debt. As Alan Greenspan once said, “children, dogs, cats, and moose are getting credit cards.”
Looking at bank lending gives a sense of scale, according to Rowena Olegario in The Engine of Enterprise of the era's rapid growth. “In the century from the 1970s to the 1970s, the ratio of bank loans to the gross domestic product was 40 to 50 percent. But afterward, bank loans grew until they were as large as the nation’s GDP” (172). Mortgages and easy debt flooded every sector of the economy. “The massive expansion of household debt can best be grasped by expressing it as a share of the nation’s GDP: it went from 100 percent of GDP in 1980 to 173 percent in 2009, or the equivalent of around $6 trillion of additional spending.” (217) Then disaster struck. Housing prices began declining, triggering a wave of defaults that contaminated mortgage-backed securities funds and threatened American GSEs. The US propped up Fannie Mae and Freddie Mac. Still, the contagion spread and sent Lehman Brothers, the world’s fourth-largest bank, into bankruptcy and threatened AIG, a major international insurance company. The US government stabilized the debt markets with the Trouble Asset Relief Program (TARP) to keep the global financial system running. It began “quantitative easing,” “purchasing huge amounts of troubled mortgage-backed securities and GSE debts as well as long-term US Treasury securities” (219).
Reform in the form of capital requirements and new accounting standards came in the subsequent decade and has been factored into credit scoring, but, as Raymond Anderson said, in general, character and obligation are no longer a factor in credit scoring: “We now have moved to the other extreme where credit scores are proxies for character—where criminal delinquents often cannot be distinguished from unfortunates affected by adverse life events based on available data.” Our entire ecosystem is in shambles because of it.
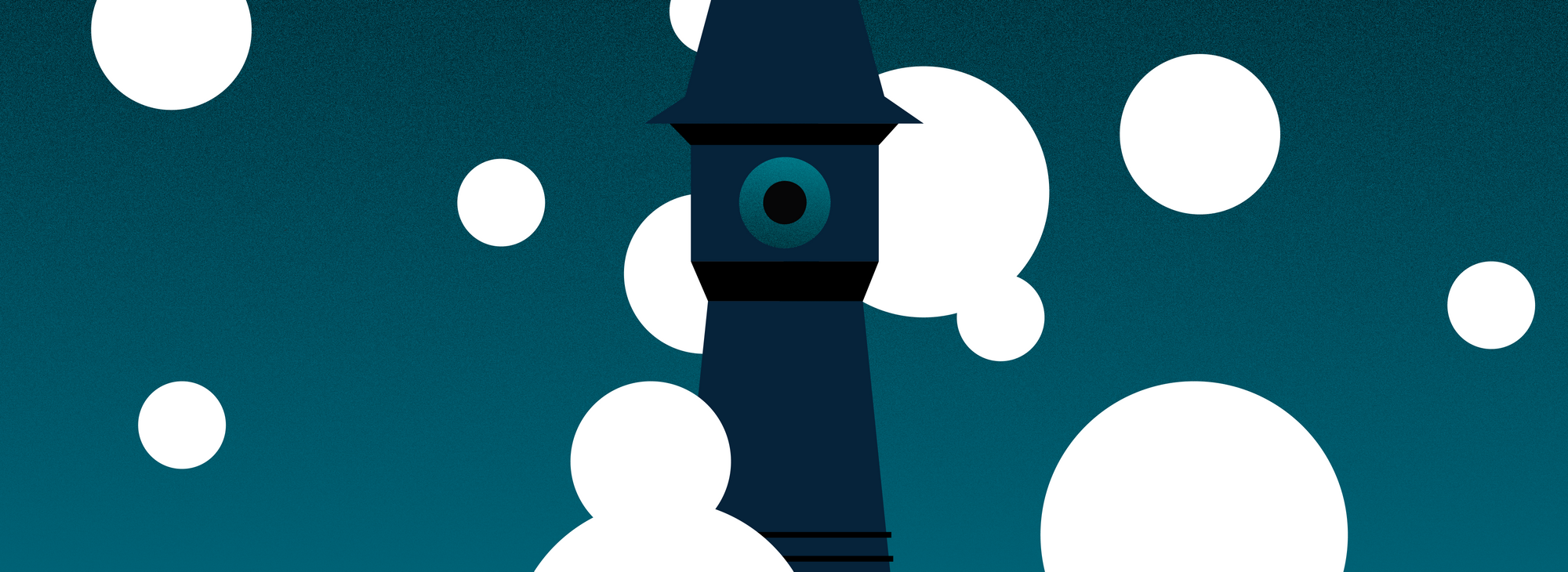
Decentralization, Scoracle networks, and the Future of Credit Scoring
Given the runaway spending to prop up lenders who had wiped out in the marketplace (the phrase “too big to fail” referring to titanic banks was popularized by then-President George W. Bush), it was no surprise a technological alternative was developed. In 2009, Bitcoin’s genesis block was mined by Satoshi Nakamoto and contains the message, “Chancellor on the brink of second bailout for banks,” referring to the day’s Times headline.
Bitcoin was a combination of peer-to-peer file network sharing and a unique method of using cryptography for reaching consensus, preventing double-spending, and making maleficence extremely expensive. In essence, it was digital cash, allowing the transfer of value between two disparate parties without the need to trust one another. This idea, and subsequent cryptocurrency developments such as Ethereum, a trustless network on which Turing complete “smart contracts” can be executed trustlessly and entirely on-chain, created an entirely new segment of decentralized finance (DeFi).
DeFi has enormous potential. All public blockchain transactions are visible, meaning all DeFi activity is auditable and transparent. It’s also accessible: anyone with a mobile phone can transact with anyone else in the world, and access sophisticated financial instruments like insurance, investing, and derivatives which can essentially level the financial playing field. Yet much of this enormous potential remains locked.
The difficulty with the trustless nature of DeFi is that it is extremely difficult to create a mature creditworthiness assessment infrastructure on the level articulated in the last three essays in this series. Without credit, credit intelligence, and credit scoring, web3 would likely remain stuck in the pre-consumer paradigm that kept economies growing at a snail’s pace before the industrial revolution. The missing ingredient to DeFi is credit scoring. The challenge will be to develop credit scoring that is as accurate and accessible as the creditworthiness assessment infrastructure that has emerged in the West without the severe drawbacks described throughout the series.
The History of Credit is optimistic: there was a movement from seeing debt as a shameful obligation and a brutal zero-sum game to a mutually beneficial relationship. There was the movement from credit being issued only to trusted networks and small segments of the population to it being available to the entire population, albeit at the expense of our collective privacy and the concentration of power into the hands of banks and bureaus, and other centralized authorities who have proven–as dozens of boom-bust cycles and the bailouts following the 2009 Credit Crunch confirm–they don’t necessarily deserve.

At Spectral Finance, we’re building a bridge between the accuracy of traditional credit scoring and a decentralized, fully accessible, auditable future. We’re building the seedbed, a set of powerful analytical tools capable of capturing the millions of DeFi, DEX, and NFT transactions on the Ethereum blockchain and using that data as the raw material for credit intelligence. Our first feature was the MACRO Score, a risk assessment score indexing seven categories to generate a single, human-readable score.
The next stage is converting that raw blockchain data into interpretable signals, making inferences akin to what credit bureaus and scoring systems do with FICO Scores, grinding insights and actionable data from the millions of transactions. Spectral’s MACRO Score, which measures a wallet’s possibility of default, is one example, but there are other valuable inferences that can be made from on-chain information in DeFi, gaming, NFTs, or retail.
Spectral’s MACRO Score took the form of a non-fungible credit token which allowed different wallets to be bundled together to preserve a measure of anonymity and to allow communities of wallet owners to combine their records allowing for the possibility of a single Score representing an entire community for example, eventually allowing a coop to be able to borrow funds without needing a bank or attorney’s assistance. Scoring is essentially the art of creating an all-encompassing model, and consolidating inferences, and again this is another area where on-chain information can be abstracted into a useful data product, akin to a FICO Score or the more customized VantageScores. Spectral is working to develop new packages of data inferences and models. The final stage of development for creating a fair, accountable, and transparent creditworthiness infrastructure is to decentralize it completely (exiting to community). For Spectral this would entail creating and incentivizing a network of on-chain oracles who would create models and data sets from the on-chain information. No single entity would be able to hoard data or provide a single source of truth the way that the bureaus and Fair Isaac came to represent.
Softening attitudes toward credit created enormous economic opportunity at the expense of privacy. DeFi has the potential to expand the benefits of easy credit to markets and create heretofore unimagined financial products and opportunities. However, the risk of creating another source of information for bureaus to hoard and feed into their models is too great to port existing scores into a new system. We're building a new financial world, why not build a better credit infrastructure while we're at it?