Spectral’s Vision for Trustless ML with On-Chain Rewards
Introducing Spectral's vision for a trustless machine learning (ML) oracle. Using zero-knowledge machine learning and other Web3 technologies allows us to move past the traditional limitations of competitive machine learning platforms.
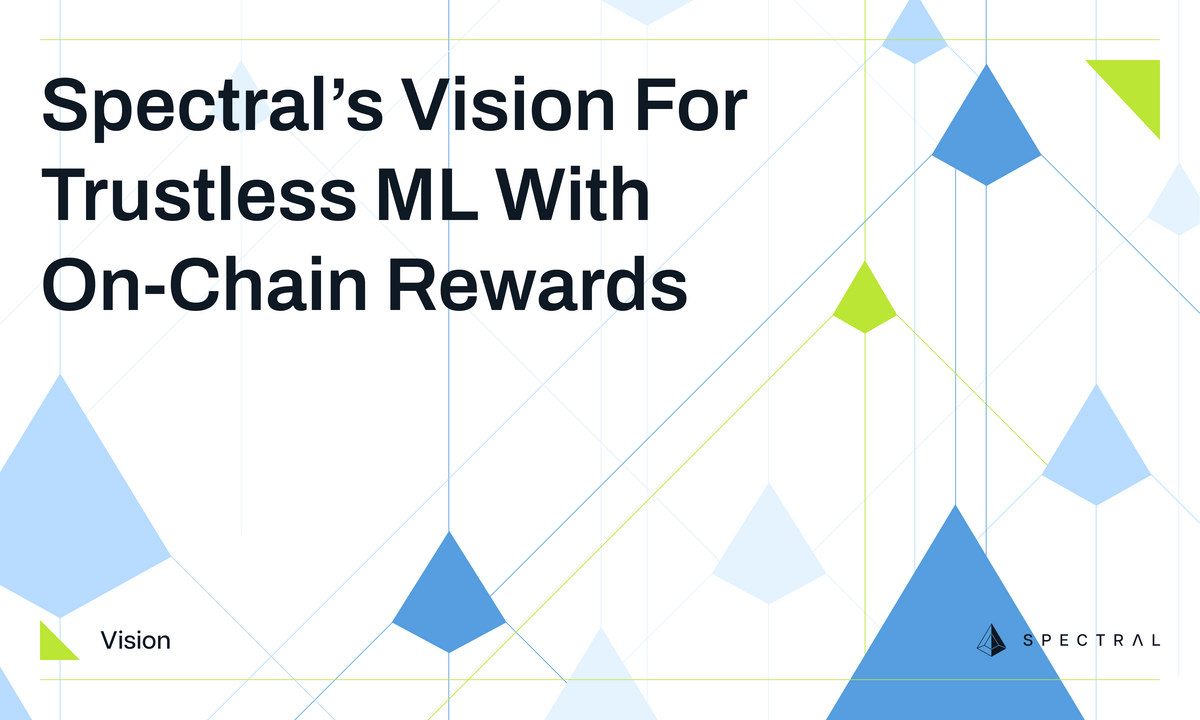
At Spectral, our long term vision is to empower the world to harness a decentralized machine learning oracle that democratizes access to high-quality, crowd-sourced, verifiable, and privacy-preserving inferences for transformative real-world impact across industries.
The Genesis of a Revolution
We are grateful to our early adopters, such as Teller, Bulla, Sardine, QuestHub, Gatekeeper, and more, for trusting us, using the MACRO Score, and providing valuable feedback. As detailed in our two-part blog series (part one, part two), and demonstrated in our simulation studies, the MACRO Score can have a significant impact on capital efficiency in the DeFi space.
While the MACRO Score was a step in the right direction, the real challenge was creating a better machine learning platform. As we delved deeper into the intricacies of Web3, and explored the potential of zero knowledge machine learning (zkML), we realized the MACRO Score was more than an on-chain credit score; it was proof of concept for a much larger vision.
In recent years, ML has quietly become a ubiquitous part of our lives. Carefully weighted algorithms sort our news feeds, recommend our movies and television shows, generate our credit scores, index our risk to insurers, detect tumors, and discover what our genes can do. They’re a product of the vast expansion of data collection and, today, bring unparalleled improvements to our lives.
These algorithms have been developed within enormous centralized institutions, putting them out of reach of the vast majority of people whose lives are directly affected by them. Mistakes are made and can be difficult or impossible for an outsider to see. In 2022, Equifax admitted a program error had garbled hundreds of thousands of credit scores. Only five years before, a security breach leaked the financial records of more than 146 million American and Canadian consumers. Outside of the United States, the Australian government was criticized for its Robodebt system, which erroneously issued thousands of debt notices to welfare recipients between 2016 to 2019. Other systems such as a British visa system in 2020, a South African medical school admissions algorithm (2019), Canada’s use of algorithms for certain legal determinations or Holland’s SyRi (System Risk Indication) have also been heavily criticized for the shortcomings of the data sets and ML models.
There is another way—A competitive ML platform could offer a truly decentralized machine learning oracle. Imagine the MACRO Score developed and perfected by a global community of data scientists, working in a system that can provide provably fair validation, while ensuring its integrity without exposing any intellectual property.
Existing competitive ML platforms offer some of these features but not all, and certainly not in the general, robust, business-ready form a credit score or admissions system would require. But with the use of zero-knowledge proofs, and other blockchain technologies, a safe, secure, equitable solution may be at hand.
The Broader Context: Unpacking the Problem Landscape
When we look back at the technological developments that defined the early 21st Century, the ones that stand out the most all distributed power from large, centralized institutions to individual experts. The combination of open source software and cloud computing allowed tech companies to explore technological frontiers and rapidly scale up to deliver their products to consumers. One of the ideas that began propagating was the idea of a crowdsourced machine learning platform.
The idea may have been inspired by distributed computing programs like SETI@home (1999), which used distributed networks to hunt for signs of alien life in radio signals or Folding@home (2000) which did the same for proteins. Both attempted to harness the power of the crowd to tackle a much larger problem. In 2002, UC Berkeley released BONIC, a general open source crowdsourcing network that relied on credits to acknowledge volunteer work, to break larger projects into much smaller tasks, and distribute them. A number of other distributed computing projects were run on the platform including climateprediction.net and Einstein@home, which predicted climate effects and neutron stars respectively.
Competitive ML platforms flipped the idea, instead of appealing to the crowd’s altruistic impulses, they offered an economic incentive, paying a bounty for whomever could best solve their problem. First there were paid distributed computing programs like Amazon’s Mechanical Turk, started in 2005, divided up large tasks and distributed them and verified and paid for them. Meanwhile, Amazon and Netflix, borrowing a format started by the ANSARI X Prize (1996) began offering large bounties. Netflix offered to pay (and eventually did pay) a million dollars to whomever could improve their recommendation algorithm by 10 percent.
Kaggle was created in 2010, offering sponsors an easy-to-use platform where machine learning modelers all over the world could accept challenges and offer solutions to win prizes and climb their leaderboards, along with excellent teaching resources and public notebooks. They were bought by Google in 2017, and spawned a number of competitors.

More than a decade later, competitive machine learning platforms are an excellent teaching tool, modelers all over the world have cut their teeth using them, and the data sets they offer are often put to use elsewhere, but few modelers stay and the results haven’t quite lived up to their full potential. Some of those reasons are structural: an open competitive platform means anyone can fork the winning model, and it’s usually impossible for modelers to share in the proceeds from those models.
Spectral counts many modelers, machine learning engineers and data scientists among its users and stakeholders. We knew that Web3 could offer a variety of solutions: Zero-knowledge machine learning (zkML) provides a method of concealing the underlying, proprietary details of a model's design and data, while mathematically verifying that modelers are only submitting inferences generated by the same models they originally committed, preserving the inferences' integrity and usefulness, while preventing theft of modelers’ intellectual property.
Streaming on-chain payments offer a way for modelers to capitalize on their work; and the flexibility provided by various crypto-economic structures allow challenge creators to sponsor challenges that are more complex than optimizing a single benchmark.
To really understand what could improve the competitive machine learning model we began a product research program, interviewing modelers from every walk of life and cataloging their reactions. What we heard has been instrumental in shaping our vision for the future of machine learning.
Data Scientists want more from their ML Platforms
The data scientists we spoke to ranged from senior credit analysts at major financial institutions and professors working at the cutting edge of the field to autodidact web3 degens scraping data directly from Etherscan to feed their models. A number of patterns emerged from our interviews.
Compensation and incentives
“A lot of people are motivated by money,” said one interviewee. “I do wanna get paid for my work,” agreed another. But they also pointed to other incentives that weren’t being well served by existing platforms, such as the potential for networking or working on a real world problem: “If I’m doing something for the public good, then [financial compensation] becomes less important.” “If I have a personal stake in something and can offer my technical skills then that’s the kind of incentive I might go for.”
Intellectual property and data privacy concerns
Modelers are increasingly wary of how their data and models are used, stored, and potentially monetized. They seek clarity on ownership and rights, a need that current platforms often neglect. “You have to do your work in a very simple manner,” said one interviewee. “Sometimes, you publish your solution, and it'll be copied."
Community and skill development
Platforms often fall short of providing enough opportunities to meet one another and develop their skills beyond a certain level. “At first I did everything within Kaggle. But I quickly needed more.” These platforms do help ideas propagate, said one interviewee: “[they] are a good place for ideas to thrive and for people to share different techniques that they are interested in.” The winner-takes-all model popular on most competitive machine learning platforms can blunt the collaboration and networking that many data scientists are looking for, however.
Our Long-Term Vision: Trustless ML with On-Chain Rewards
Imagine earning big by solving the world’s most pressing problems, while optimizing your ML skills and domain-specific expertise. Then, imagine having immutable ownership and protection of that hard work, along with the support of a collaborative community. Finally, imagine intelligence decentralized—transforming the vast expanse of ML into a shared commons where insights are accessible to all.

At Spectral, our goal is to empower the world with a trustless ML oracle that can bring to life the vision above. The collective intelligence of engineers, data scientists, and ML enthusiasts—like you—have an indispensable role to play.
To give you a chance to participate in what we’re building, we’re excited to be opening up our first ML modeling challenge. We designed this challenge around decentralized finance (DeFi), an area we at Spectral are deeply familiar with. And our intent is to put the winning models to immediate use to solve for DeFi’s increasingly complex and impactful insight gaps. We’re offering a $100,000 bounty to model developers whose submissions can exceed the performance benchmark we’re setting for our inaugural competition—with an additional 85% share generated through any ongoing, real-world application of those models. We will announce details of the challenge this week—sign up for our waitlist and be the first to know when new data, learning materials and other information drops.
And that’s just the beginning. As we grow our platform, we plan to partner with carefully selected sponsors to post a variety of additional challenges that call on new skill-sets and subject matter knowledge, providing you with opportunities to expand your capabilities - and potentially get paid handsomely to do so. We will also incorporate new, breakthrough technologies like distributed networking, verifiable computation, and Zero Knowledge Machine Learning (zkML), evolving our platform so that you will benefit not only from automated, tamper-proof evaluation mechanisms, but other industry-leading features to keep your models private and your payouts seamless. Excited to learn more? Connect now with other data scientists and the Spectral team.
To us, building a decentralized ML oracle is our response to the open source movement, where collaboration and transparency transformed industries. We believe scalable, on-chain ML is a revolutionary paradigm shift, and we invite you to join us in making that happen.